The telecommunications industry faces distinct challenges that require innovative solutions to enhance network performance, customer experience, and operational efficiency. Next-generation technologies such as Artificial Intelligence (AI), IoT sensors, Machine Learning (ML), automation, chatbots, and Large Language Models (LLMs) can address these challenges effectively. This article outlines the key challenges in the telecommunications industry, tailored IT solutions, and detailed case studies with cost-benefit analysis, including real-time data integration and analytics.Â
In-House Engineers
Customer Satisfaction
We Have Completed
Client’s Reviews
Key Challenges in the Telecommunications Industry
- Network Optimization and Performance
- Customer Service and Engagement
- Fraud Detection and Prevention
- Operational Efficiency
- Data Management and Security
- Predictive Maintenance
Tailored IT Solutions
- AI and Machine Learning for Network Optimization
- Chatbots and LLMs for Customer Service
- AI and ML for Fraud Detection
- Automation for Operational Efficiency
- IoT for Data Management and Security
- Predictive Maintenance using AI and ML
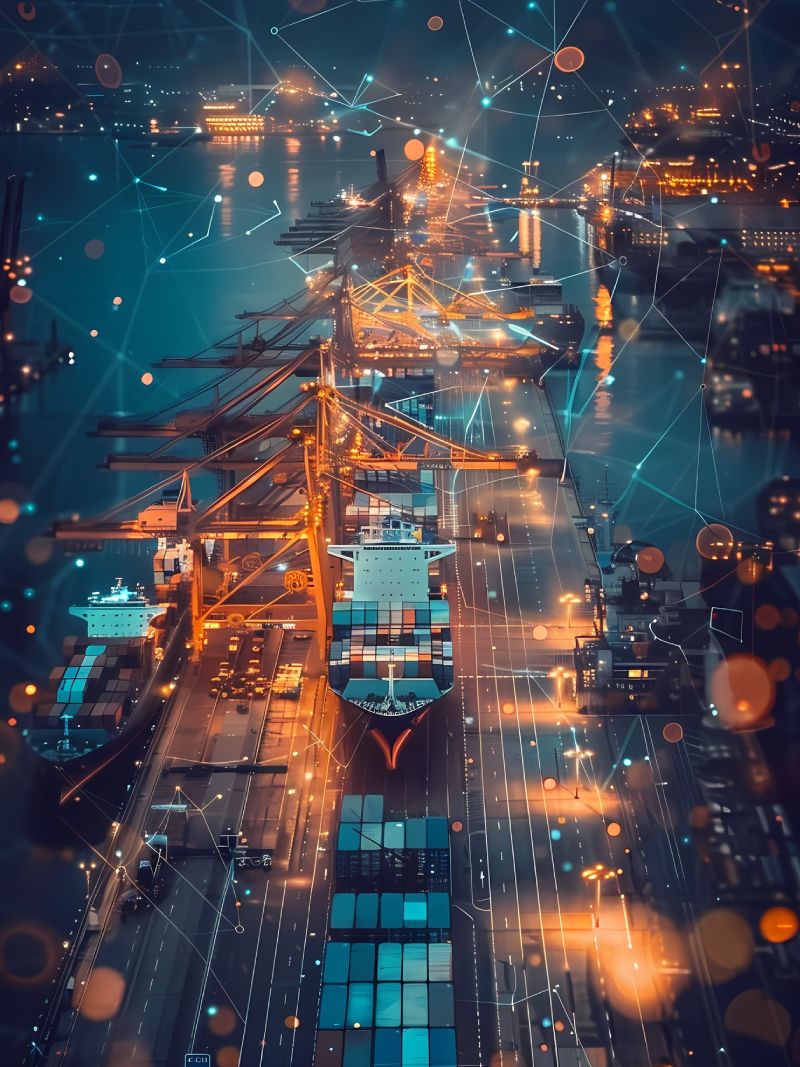
AI and Machine Learning for Network Optimization
Challenge
- Ensuring optimal network performance and minimizing downtime.
Solution
- Implement AI and ML algorithms to analyze network data and optimize performance in real-time.
Cost-Benefit Analysis
- Initial Cost: $1,500,000
- Annual Maintenance: $300,000
- Annual Savings: $1,200,000 (from improved performance and reduced downtime)
- ROI Period: 1.5 years
Case Study: AT&T’s AI-Driven Network Optimization
Implementation
- AI and ML models to monitor and optimize network performance.
Cost
- Initial setup cost of $1,500,000, with annual maintenance of $300,000.
Benefit
- Improved network performance by 35%.
- Reduced downtime by 25%.
- Enhanced customer satisfaction.
Chatbots and LLMs for Customer Service
Challenge
- Providing efficient and personalized customer support.
Solution
- Develop chatbots and LLMs to handle customer inquiries, provide account information, and assist with troubleshooting.
Cost-Benefit Analysis
- Initial Cost: $600,000
- Annual Maintenance: $120,000
- Annual Savings: $500,000 (from reduced support costs and improved customer satisfaction)
- ROI Period: 1.5 years
Case Study: Verizon’s AI Chatbot for Customer Support
Implementation
- AI-powered chatbot for customer support and engagement.
Cost
- Initial setup cost of $600,000, with annual maintenance of $120,000.
Benefit
- Improved customer satisfaction by 30%.
- Reduced call center volume by 40%.
- Enhanced customer interaction and support efficiency.
AI and ML for Fraud Detection
Challenge
- Detecting and preventing fraudulent activities in real-time.
Solution
- Implement AI and ML algorithms to analyze transaction patterns and identify fraudulent activities.
Cost-Benefit Analysis
- Initial Cost: $800,000
- Annual Maintenance: $160,000
- Annual Savings: $700,000 (from reduced fraud losses)
- ROI Period: 1.5 years
Case Study: T-Mobile’s AI Fraud Detection System
Implementation
- AI and ML models to monitor and analyze transactions for signs of fraud.
Cost
- Initial setup cost of $800,000, with annual maintenance of $160,000.
Benefit
- Reduced fraud losses by 45%.
- Enhanced transaction security.
- Improved customer trust.
Automation for Operational Efficiency
Challenge
- Enhancing operational efficiency and reducing manual labor costs.
Solution
- Implement automation solutions for various operational processes such as billing, monitoring, and control.
Cost-Benefit Analysis
- Initial Cost: $900,000
- Annual Maintenance: $180,000
- Annual Savings: $800,000 (from reduced operational costs and increased efficiency)
- ROI Period: 1.5 years
Case Study: Vodafone’s Automated Operations Platform
Implementation
- Automation of operational processes using RPA (Robotic Process Automation) tools.
Cost
- Initial setup cost of $900,000, with annual maintenance of $180,000.
Benefit
- Reduced operational costs by 30%.
- Increased efficiency and accuracy of production tasks.
- Improved staff productivity.
IoT for Data Management and Security
Challenge
- Managing and securing vast amounts of data generated by network operations.
Solution
- Deploy IoT sensors to monitor data flow and ensure data security in real-time.
Cost-Benefit Analysis
- Initial Cost: $700,000
- Annual Maintenance: $140,000
- Annual Savings: $600,000 (from reduced data breaches and improved security)
- ROI Period: 1.5 years
Case Study: BT’s IoT-Enabled Data Security System
Implementation
- IoT sensors for real-time monitoring of data flow and security.
Cost
- Initial setup cost of $700,000, with annual maintenance of $140,000.
Benefit
- Improved data security by 40%.
- Reduced data breaches and associated costs.
- Enhanced overall data management.
Predictive Maintenance using AI and ML
Challenge
- Reducing equipment downtime and maintenance costs
Solution
- Implement AI and ML algorithms to predict equipment failures and schedule maintenance proactively.
Cost-Benefit Analysis
- Initial Cost: $800,000
- Annual Maintenance: $160,000
- Annual Savings: $700,000 (from reduced maintenance costs and improved uptime)
- ROI Period: 1.5 years
Case Study: Nokia’s Predictive Maintenance System
Implementation
- AI and ML models to analyze equipment data and predict failures.
Cost
- Initial setup cost of $800,000, with annual maintenance of $160,000.
Benefit
- Reduced maintenance costs by 20%.
- Increased equipment uptime by 15%.
- Enhanced operational efficiency and reliability.
Conclusion
Integrating AI, IoT, ML, automation, chatbots, and LLMs in the telecommunications industry addresses critical challenges and opens up new opportunities for growth and efficiency. The detailed case studies and cost-benefit analyses demonstrate the significant potential of these technologies to enhance network optimization, customer service, fraud detection, operational efficiency, data management, and predictive maintenance. By leveraging these next-generation solutions, the telecommunications industry can become more resilient, efficient, and future-ready, ultimately leading to improved operational performance and customer satisfaction.Â