The agriculture industry faces numerous challenges that require innovative solutions. Next-generation technologies such as Artificial Intelligence (AI), IoT sensors, Machine Learning (ML), automation, chatbots, and Large Language Models (LLMs) can address these challenges effectively. This article outlines the key challenges in agriculture, tailored IT solutions, and detailed case studies with cost-benefit analysis, including real-time data integration and analytics.Â
In-House Engineers
Customer Satisfaction
We Have Completed
Client’s Reviews
Key Challenges in Agriculture
- Climate Change and Weather Prediction
- Resource Management
- Crop Monitoring and Health
- Soil Health Monitoring
- Supply Chain Management
- Crop Monitoring and Health
Tailored IT Solutions
- AI and Machine Learning for Weather Prediction
- IoT Sensors for Resource Management
- AI and ML for Crop Health Monitoring
- IoT and Automation for Soil Health
- Blockchain and IoT for Supply Chain Management
- Automation and Robotics for Labor Efficiency
- Chatbots and LLMs for Farmer Support
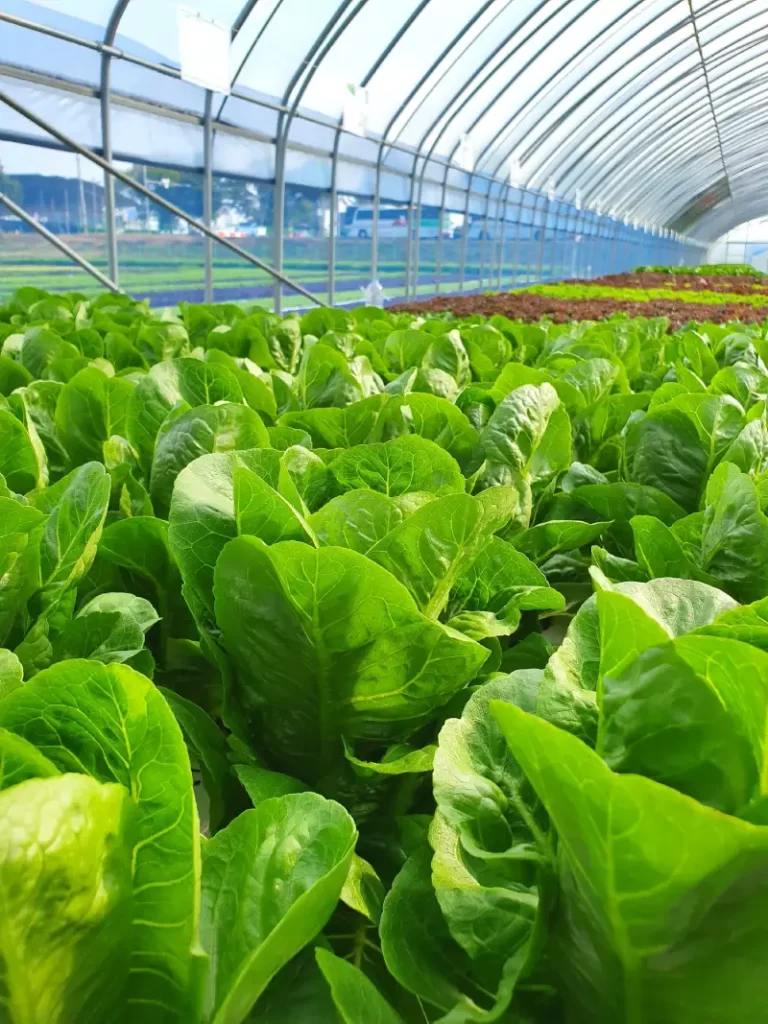
AI and Machine Learning for Weather Prediction
Challenge
- Unpredictable weather patterns affecting crop yields and farming schedules.
Solution
- Develop AI models that analyze historical weather data and predict future weather patterns with high accuracy.
Cost-Benefit Analysis
- Initial Cost: $50,000
- Annual Maintenance: $10,000
- Annual Savings: $30,000 (from reduced losses and increased yield)
- ROI Period: 2 years
Case Study: IBM Watson Decision Platform for Agriculture
Implementation
- AI-based weather prediction models integrated with real-time weather data.
Cost
- Initial setup cost of $50,000, with annual maintenance of $10,000.
Benefit
- Improved crop yield by 15%.
- Reduced losses from weather-related damages by 20%.
- ROI achieved within 2 years due to increased productivity.
AI and ML for Crop Health Monitoring
Challenge
- Early detection of diseases, pests, and nutrient deficiencies.
Solution
- Use AI and ML algorithms to analyze images from drones and satellites for early detection.
Cost-Benefit Analysis
- Initial Cost: $30,000
- Annual Maintenance: $5,000
- Annual Savings: $20,000 (from reduced losses and control costs)
- ROI Period: 1.5 years
Case Study: PEAT Plantix App
Implementation
- AI-based image analysis for disease detection.
Cost
- Initial setup cost of $30,000, with annual maintenance of $5,000.
Benefit
- Reduced crop loss by 20%.
- Early intervention saved 15% on pest control costs.
- Improved overall crop health, leading to a 10% yield increase.
IoT Sensors for Resource Management
Challenge
- Efficient use of water, fertilizers, and pesticides.
Solution
- Implement IoT sensors to monitor soil moisture, temperature, and nutrient levels in real-time.
Cost-Benefit Analysis
- Initial Cost: $75,000
- Annual Maintenance: $15,000
- Annual Savings: $40,000 (from reduced resource usage and increased yield)
- ROI Period: 2.5 years
Case Study: John Deere Precision Agriculture Solutions
Implementation
- IoT sensors deployed across fields to monitor resource usage.
Cost
- Initial setup cost of $75,000, with annual maintenance of $15,000.
Benefit
- Water usage reduced by 25%.
- Fertilizer usage optimized, leading to a 20% cost reduction.
- Increased crop yield by 10%.
IoT and Automation for Soil Health
Challenge
- Soil degradation and nutrient depletion.
Solution
- Deploy IoT-enabled soil sensors and automate the application of fertilizers.
Cost-Benefit Analysis
- Initial Cost: $60,000
- Annual Maintenance: $12,000
- Annual Savings: $35,000 (from increased yield and reduced fertilizer usage)
- ROI Period: 2 years
Case Study: PEAT Plantix App
Implementation
- IoT sensors to monitor soil conditions and automated fertilization systems.
Cost
- Initial setup cost of $60,000, with annual maintenance of $12,000.
Benefit
- Improved soil health resulting in a 15% increase in crop yield.
- Reduced fertilizer usage by 20%.
- Enhanced sustainability practices.
Blockchain and IoT for Supply Chain Management
Challenge
- Efficient logistics and storage solutions to reduce post-harvest losses.
Solution
- Utilize blockchain for transparent supply chain tracking and IoT sensors for real-time monitoring of produce conditions.
Cost-Benefit Analysis
- Initial Cost: $100,000
- Annual Maintenance: $20,000
- Annual Savings: $50,000 (from reduced losses and increased market price)
- ROI Period: 2.5 years
Case Study: Agri Digital Blockchain Platform
Implementation
- Blockchain for supply chain tracking and IoT sensors for monitoring.
Cost
- Initial setup cost of $100,000, with annual maintenance of $20,000.
Benefit
- Reduced post-harvest losses by 25%.
- Increased transparency and trust in the supply chain.
- Enhanced quality control, leading to a 10% increase in market price.
Automation and Robotics for Labor Efficiency
Challenge
- Skilled labor shortages and high labor costs.
Solution
- Implement robotic systems for planting, weeding, and harvesting crops.
Cost-Benefit Analysis
- Initial Cost: $200,000
- Annual Maintenance: $40,000
- Annual Savings: $80,000 (from reduced labor costs and increased productivity)
- ROI Period: 2.5 years
Case Study: Naio Technologies Agricultural Robots
Implementation
- Agricultural robots for weeding and harvesting.
Cost
- Initial setup cost of $200,000, with annual maintenance of $40,000.
Benefit
- Reduced labor costs by 30%.
- Increased efficiency and productivity by 20%.
- Improved operational consistency and reliability.
Chatbots and LLMs for Farmer Support
Challenge
- Timely access to agricultural advice and information.
Solution
- Develop chatbots and LLMs to provide farmers with instant access to advice, weather updates, and market prices.
Cost-Benefit Analysis
- Initial Cost: $20,000
- Annual Maintenance: $5,000
- Annual Savings: $15,000 (from increased productivity and reduced advisory costs)
- ROI Period: 1.5 years
Case Study: Microsoft AI Chatbot for Indian Farmers
Implementation
- AI-powered chatbot for personalized farmer support.
Cost
- Initial setup cost of $20,000, with annual maintenance of $5,000.
Benefit
- Improved decision-making, leading to a 10% increase in productivity.
- Reduced reliance on traditional advisory services.
- Enhanced access to market and weather information.
Conclusion
Integrating AI, IoT, ML, automation, chatbots, and LLMs in the agriculture industry addresses critical challenges and opens up new opportunities for growth and sustainability. The detailed case studies and cost-benefit analyses demonstrate the significant potential of these technologies to enhance productivity, reduce costs, and ensure sustainable farming practices. By leveraging these next-generation solutions, the agriculture industry can become more resilient, efficient, and future-ready.